Photonics2D#
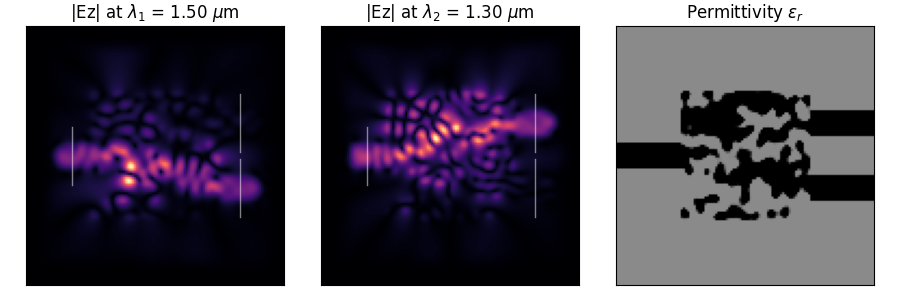
Version |
0 |
Design space |
|
Objectives |
total_overlap: ↑ |
Conditions |
lambda1: 1.5 lambda2: 1.3 blur_radius: 2 |
Dataset |
|
Import |
|
Photonic Inverse Design 2D Problem (Wavelength Demultiplexer).
Problem Description#
Optimize a 2D material distribution (rho
) to function as a wavelength
demultiplexer, routing wave with lambda1
to output 1 and lambda2
to output 2. The
design variables represent material density which is converted to permittivity
using filtering and projection.
Design space#
2D tensor rho
(num_elems_x, num_elems_y) with values in [0, 1], representing material density.
Stored as design_space
(gymnasium.spaces.Box).
Objectives#
total_overlap
: Objective to maximize, defined asoverlap1 * overlap2
. Higher is better. This is corresponds to the overlap in the target electrical fields with the desired demultiplexing locations. Note that botsimulate
andoptimize
subtract a small material penalty (total_overlap - penalty
) to avoid multiple equivalent local optima, but this penalty is small relative to the overlap objective.
Conditions#
These are designed as user-configurable parameters that alter the problem definition.
Default problem parameters that can be overridden via the config
dict:
lambda1
: The first input wavelength in μm (default: 1.5 μm).lambda2
: The first input wavelength in μm (default: 1.3 μm).blur_radius
: Radius for the density blurring filter (default: 2). Higher values correspond to larger elements, which could possibly be more manufacturable.num_elems_x
: Number of grid cells in x (default: 120).num_elems_y
: Number of grid cells in y (default: 120).
In practice, for the dataset loading, we will keep num_elems_x
and num_elems_y
to set
values for each dataset, such that different resolutions correspond to different
independent datasets.
Optimization Parameters#
Note: These are advanced parameters that alter the optimization process – we do not recommend changing these if you are only using the library for benchmarking, as it could make results less reproducible across papers using this problem.)
num_optimization_steps
: Total number of optimization steps (default: 300).step_size
: Adam optimizer step size (default: 1e-1).penalty_weight
: Weight for the L2 penalty term (default: 1e-2). Larger values reduce unnecessary material, but may lead to worse performance if too large.eta
: Projection center parameter (default: 0.5). There is little reason to change this.N_proj
: Number of projection applications (default: 1). Increasing this can help make the design more binary.N_blur
: Number of blur applications (default: 1). Increasing this smooths the design more.initial_beta
: Initial beta for the optimization continuation scheme (default: 1.0).save_frame_interval
: Interval for saving intermediate design frames during optimization. If > 0, saves a frame everysave_frame_interval
iterations to theopt_frames/
directory. Default is 0 (disabled).
Internal Constants#
Note: These are not typically changed by users, but provided here for technical reference
dl
: Spatial resolution (meters) (default: 40e-9).Npml
: Number of PML cells (default: 20).epsr_min
: Minimum relative permittivity (default: 1.0).epsr_max
: Maximum relative permittivity (default: 12.0).space_slice
: Extra space for source/probe slices (pixels) (default: 8).
Simulator#
The simulation uses the ceviche
library’s Finite Difference Frequency Domain (FDFD)
solver (fdfd_ez
). Optimization uses ceviche.optimizers.adam_optimize
with
gradients computed via automatic differentiation (autograd
).
Dataset#
This problem currently provides one dataset corresponding to resolution of 120x120, are available on the Hugging Face Datasets Hub.
v0#
Fields#
Each dataset contains:
lambda1
: The first input wavelength in μm.lambda2
: The second input wavelength in μm.blur_radius
: Radius for the density blurring filter (pixels).optimal_design
: The optimal design density array (shape num_elems_x, num_elems_y).optimization_history
: A list of objective values from the optimization process (field overlap minus penalty, where higher is better) – This is for advanced use.
Creation Method#
To generate a dataset for training, we generate (randomly, uniformly) swept over the following parameters:
\(\lambda_1 \in [0.5\mu m, 1.25\mu m]\) =
lambda1
=rng.uniform(low=0.5, high=1.25, size=20)
– This corresponds roughly to a portion of the visible spectrum up to near-infrared.\(\lambda_2 \in [0.75\mu m, 1.5\mu m]\) =
lambda2
=rng.uniform(low=0.75, high=1.5, size=20)
– This corresponds roughly to a portion of the visible spectrum up to near-infrared.\(r_{blur}\) =
blur_radius
=range(0, 5)
Citation#
This problem is directly refactored from the Ceviche Library: https://github.com/fancompute/ceviche and if you use this problem your experiments, you can use the citation below provided by the original library authors:
@article{hughes2019forward,
title={Forward-Mode Differentiation of Maxwell's Equations},
author={Hughes, Tyler W and Williamson, Ian AD and Minkov, Momchil and Fan, Shanhui},
journal={ACS Photonics},
volume={6},
number={11},
pages={3010--3016},
year={2019},
publisher={ACS Publications}
}
Lead#
Mark Fuge @markfuge